How fast the development from assisted to fully automated vehicles will progress is uncertain. One crucial factor here is the reliability with which a vehicle can navigate in its surroundings and react to unforeseeable incidents. Our group at the Max Planck Institute for Intelligent Systems showed that methods for motion analysis based on deep neural networks – likely components in future autonomous vehicles – can be confused by small patterns designed to “attack” these networks.
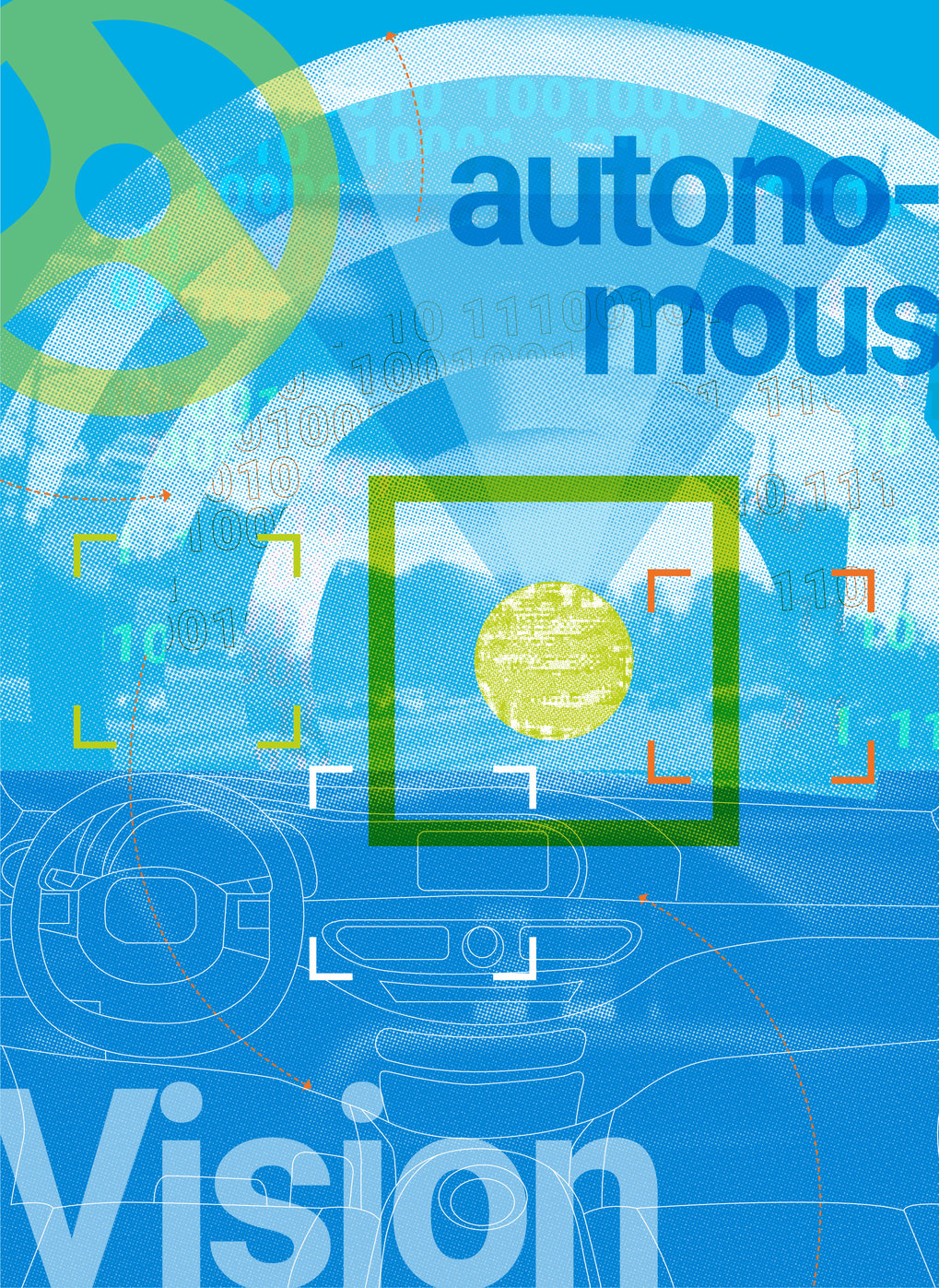
Text: Michael Black
Self-driving or semi-autonomous cars perceive their surroundings with different sensors. To analyse a scenario, manufacturers use, amongst others, optical flow, the two-dimensional motion of pixels between video frames. This is used in robots, in medicine, in special effects and in navigation, to name but a few examples.
The optical flow describes the various movements in a scene that are perceived by humans with their eyes and cars with on-board cameras. If you drive or walk somewhere, static objects such as trees, houses or pylons appear to drift in the opposite direction. The speed of movement allows us to judge the distances to the objects, amongst other things: Whereas a nearby tree rapidly disappears behind us, distant objects such as clouds or mountains appear to be standing still. We also see people or animals moving on their own.
In order to analyse the various movements, the onboard cameras record numerous images of the scene in rapid succession; a computer deduces the movement of the individual objects from the differences between the images with complex mathematical methods. It calculates a speed vector for each pixel that indicates how fast and in what direction the world sur face at the pixel moves through the image. One important aspect here is that both the movement of the vehicle and the movement of the surrounding objects, persons or other cars, cause an optical flow. The on-board computer thus has to be able to distinguish its own movement from that of other objects – a very complicated task.
Easy to produce colour patterns can disturb motion analysis
The latest progress in machine learning has led to faster and better methods to calculate such movement. In a joint project involving our Department for Perceptive Systems at the Max Planck Institute for Intelligent Systems in Tübingen and the Autonomous Vision research group of the University of Tübingen, we have demonstrated that these kinds of methods are susceptible to carefully constructed attacks: if, for example, a simple, colourful pattern appears in the scene, either by accident or purposefully positioned in the image data by a hacker attack. Even if the pattern does not move, it can lead to incorrect calculations by deep neural networks, as are currently widely used to calculate optical flow – the network suddenly calculates that large parts of the scene are moving in the wrong direction. Sometimes the blotch of colour can even disrupt the complete system. Such a blackout could be very risky.
The danger that existing vehicles currently available on the market are affected is low. Nevertheless, to be on the safe side, we informed a number of car manufacturers who are currently developing self-driving models. The topic of attacking neural networks is actively discussed at the leading conferences on machine vision, but we are the first to show that optical flow networks can be attacked. The goal of our project was to warn manufacturers of self-driving vehicles of the potential threat and to develop new methods that are robust to attack. Our work can help manufacturers to train their systems to withstand such disturbances.
To this end, we built five colour patches to attack these systems. It turns out to be relatively easy to come up with such patterns with a few hours of computation. We positioned these colour patterns at random points in a scene during our test runs. To our great surprise, it was very easy to disturb all five neural networks. In our test, even a small patch, making up less than one percent of an overall image, was enough to confuse the system in such a manner as to affect half of the image area. And the larger the patch, the more disastrous the consequences. The colour patches are thus very effective.
We used these patches to analyse what was happening inside these networks and found systematic biases in the networks that people were unaware of. These neural networks are loosely inspired by the way our brain works. Incoming data is analysed in the network with weights and simple computations. The system’s weights are trained so that the network learns to output the correct motion of the scene. If the network makes mistakes, this can be compared to optical illusions that can also trick the human eye.
The neural network itself is unable to change the prioritisations it has been taught, which can lead to misjudgements. However, it should be possible to retrain it so that it is no longer tricked by these kinds of illusions.
Reliable neural networks will make autonomous driving safer
The fact that neural networks still require improvement was demonstrated by a very simple test. We showed the system two identical images. Although there was no movement or change in either of them, the network identified a difference. This should not happen. And these problems show that optical flow networks are not yet sufficiently mature for autonomous vehicles. Our research work should help raise awareness of this problem.
If neural networks are reliable, they will make autonomous driving safer. This will also be aided by cars using not only cameras but also other sensors to “find their way around”. On the other hand, on-board computers in cars should be able to analyse street scenarios more easily when more autonomous vehicles are on the roads that can communicate with each other. In this case, a car is not only dependent on the signals of its own sensors, but also receives data on its position and speed from other vehicles. We are convinced that autonomous driving can make road traffic safer despite the technical weakness that we are disclosing here. After all, human error is still the cause of 90 per cent of all accidents.