What if a musically talented pianist is asked to play a popular piece for a wedding ceremony? But she has a challenging problem: The musical score cannot be found online or in stores, and nobody she knows is able to musically transcribe.
This is a job for the Hakubi Center for Advance Research, Kyoto University. A team led by Eita Nakamura, Kazuyoshi Yoshii, and Kentaro Shibata has been improving their machine learning technology for automatically and accurately transcribing multi-tone, or polyphonic, musical scores from audio data of piano performances.
In music transcription, a rigorously trained expert listens to a musical performance and painstakingly transcribes it onto sheet music. It demands high intelligence that recognizes and codes complex combinations of pitches and rhythms.
Transcribing a monophonic tune like the English “ABC” song is simple, but polyphonic music contains multiple tones played simultaneously and huge search space with the added challenge of differentiating individual pitches from a sound mixture.
Doing it by ear is tough enough. Designing a computer to execute the same task is at a whole new level of technical sophistication. Thus, this challenge has been approached as two-split problems, namely multipitch detection and rhythm quantization.
In multipitch detection, the properties of a polyphonic audio signal are analyzed for fundamental frequencies sounding at a particular point in time. Multipitch sounds are found not only in our music but also in a song sung by multiple birds or a speech given by multiple speakers simultaneously.
“The generated transcriptions demonstrate the potential for practical applications, such as assisting human transcribers to enhance musical performance,” notes Nakamura.
In rhythm quantization, the onset time and duration of each musical note are analyzed for symbolic notation, for example, a 16th note on the second beat of the 15th measure. Since all musical performances have different temporal fluctuations, symbolic notation is necessary for musicians to correctly recognize the musical structure. What for musicians is an incorrectly notated musical score is what for a grammarian a randomly punctuated piece of text resembles.
Recent advancements in multipitch detection and rhythm quantization have seen a crescendo of progress in music information processing.
The Kyoto University team’s study has two parts. The first looks at how well multipitch detection and rhythm quantization methods can be integrated, using classical music and popular music data for systematic evaluations. They used a deep neural network, also known as DNN, that estimates the pitches, intensities, and the presence of musical frequencies in the input audio signal.
Mathematical models of musical performance and scores were also instrumental in utilizing their statistical characteristics for accurate rhythm quantization.
The second part is a more theoretical analysis of the principles for correctly estimating global musical characteristics, such as tempo, meter, and bar lines, with statistical patterns in musical score data. The ultimate purpose is for improving the accuracy or integrity of the transcribed audio data.
The team’s method demonstrates a significant reduction of more than 50% in transcription errors compared to previous methods. The method improves transcription by means of statistically capturing the relationship between musical notes in a given input audio signal.
“As a result,” says Nakamura, “we succeeded in generating musical scores that can partly be used for performance while assisting human transcribers.” Examples of such transcription results can be viewed on .
But there is also a caveat regarding other musical aspects. Nakamura acknowledges that the current technology is not up to speed with the demand by music experts for high-quality transcription.
Deeper knowledge of music needs to be computationally formalized and larger amounts of data will need to be analyzed so that a wider profile of musical elements, including dynamic markings and ornamentation symbols such as arpeggios and trills, can also be automatically transcribed.
The potential for the advancement of automatic music transcription reaches beyond into other areas of application that involve big-data analysis with implications for further developments in various fields of science as well as the humanities.
The team recognizes that the transcription method being studied and developed has arrived at a finer point of convergence of scientific intelligence and the arts. Their success thus far marks a milestone in practical information technology.
Nakamura concludes, “We hope that more people will become interested in the vast potential of multidisciplinary research and appreciate its significance in how technology relates to society.”
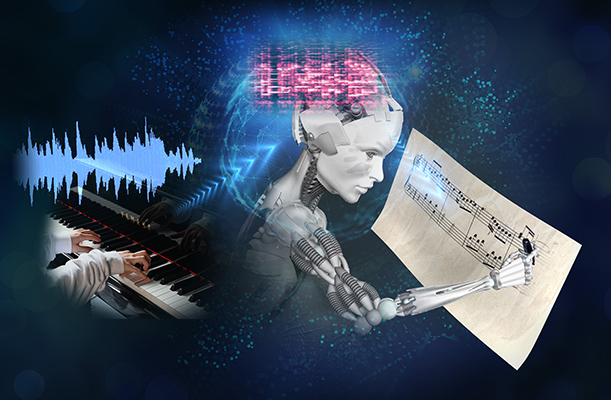